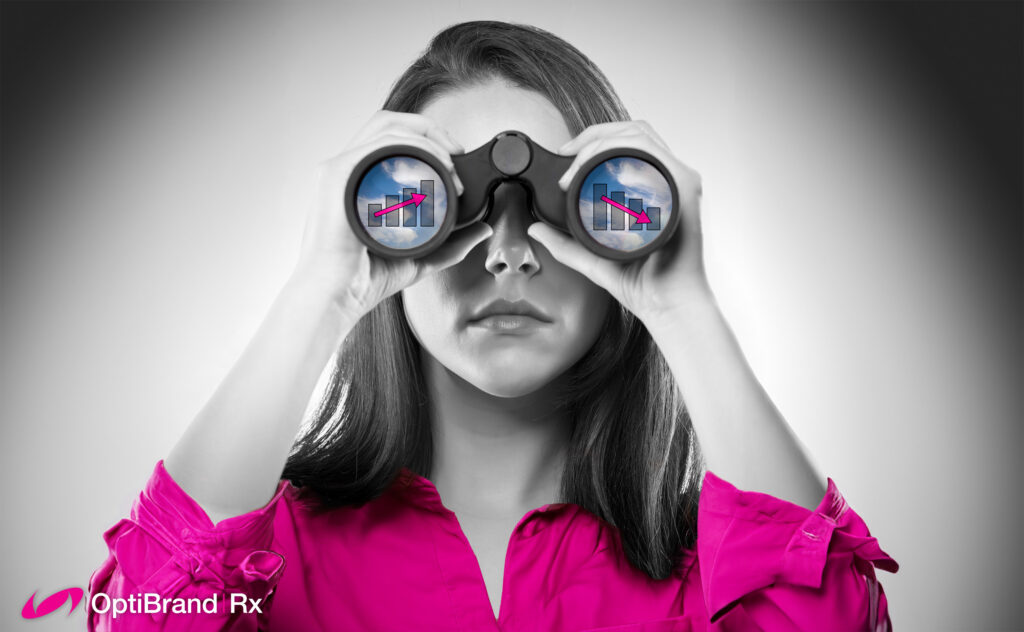
Demand studies are a keystone of pharma primary market research (PMR) plans. Whether you refer to them as demand studies, share assessments, or TPP conjoints, the fundamentals remain the same. In short, organizations are seeking information that will inform uptake, peak share potential, and competitive risk. These considerations are vital for refining forecasts and making crucial commercial decisions throughout the development process of a pharmacologic asset.
The scope of these studies varies from straightforward quantitative assessments that involve share allocations based on static TPP stimuli, to more intricate conjoint analyses that work to tease apart what a physician says they will do when making a prescribing decision versus what truly motivates them. This latter type of study is the gold standard for what teams should be using as they pencil in timelines and budgets. This more complex, but exponentially more valuable approach, is what this month’s StrategyTip® will examine.
Which conjoint approach does OptiBrand use?
There are only a handful of mathematical approaches to use in order to conduct conjoint-based studies. The one we see employed most often in healthcare market research is some derivative of Richard M. Johnson’s “Tradeoff Analysis” and “Adaptive Conjoint Analysis”. This approach mathematically dissects human decision making by forcing respondents to tradeoff conflicting criteria. This is the foundation for Sawtooth Software, which is the backbone of how a lot of market research houses conduct complex quant for big pharma today—whether they tell you or not.
In its most simplistic applications, it may be used to test levels of one’s own product profile—for example, the utility value of delivering a +4.0 month mPFS benefit vs. a +5.0 month mPFS benefit. In more complex applications, it would do the above, but also in context competitors entering the market, order of entry, and the data those competitors may or may not have at the time of their respective launches. It is effective, proven across categories, and has stood the test of time.
This all sounds great, until you realize that centralizing the power of tradeoff-based conjoint to Sawtooth and other software-as-a-service (SaaS) companies forces a tradeoff of its own: a tradeoff to you, the market researcher. If you’ve gone through the process before, inevitably you’ve been told that you have too many variables, too many levels, or too many competitors—and we didn’t even mention patient types. The reality of pharma in 2023 is that teams are being asked to deliver more with less. To extract maximum value out of every study.
While Sawtooth Software and similar have been staples to pharma market research studies, they were developed to inform product design decisions for consumer goods.
That is why OptiBrand has developed, Demand+.
So, what makes Demand+ different?
Demand studies are perfect the example of where deviating too far from the norm can impede cross-functional alignment. The pressure placed on this form of research, as well as the weight placed on the results, calls for improving upon industry-accepted standards. In the case of OptiBrand, we didn’t create an alternative to the proverbial wheel—we improved upon the design and usability of what has been proven time and time again.
Developed and refined by Melissa Tallman, Ph.D., our EVP of Research and Analytics, Demand+ was created to optimize how the power of adaptive choice-based conjoint is applied specifically in pharmaceutical market research.
1. Expanded Variables and Answer Choices
The optimal demand study needs to deliver layers of value, accounting not only for scenarios for how our own clinical program may unfold, but also scenarios for new entrants to the category and how their product profiles/TPPs take shape. Demand+ empowers market researchers by lifting the limitations of how many variables and levels can be tested simultaneously.
Beyond accounting for complex scenarios for how competition and preference within a category will evolve, the power of our in-house algorithm also lifts the limitation on the number of possible comparisons in the current competitive landscape. Across therapeutic areas, treatment landscapes are becoming more and more crowded. Not being limited in the number of competitors to which a prescriber can allocate share allows our studies to provide the most realistic current and future consideration sets for HCPs.
Lastly, having our own algorithm affords us the ability to easily create exceptions to rules within the model without compromising its statistical integrity. Gone are the days where market researchers are forced to test every permutation of their variables & levels, even the ones that don’t quite make sense. For example, assume a hypothetical future scenario that lists Product X with the lowest efficacy of the new entrants presented, but with the strongest tier NCCN recommendation—we can code in rules to the model that don’t allow these impossible scenarios.
2. Testing Multiple Product Scenarios Against Multiple Patient Types
Historically, these types of studies are often limited to either variability in product scenarios or variability in which patient type treatment is being prescribed to. Our approach enables you to explore both simultaneously.
We do not limit the number of patients types that a physician can consider for these complex future product and competitive scenarios. HCPs can allocate share to a number of different patient types as defined by you, the client.
Then, during the analysis and model building phase, our statisticians will work with epidemiology data or other primary and secondary sources to factor in prevalence of each patient type to further refine the size of the opportunity. The result is a comprehensive model that provides targeted peak share in several patient types, as well as a prevalence-corrected categorical share. The prevalence-corrected categorical share is a far more accurate estimation than the traditional broad allocation.
3. No One-Size-Fits-All Approach to Discounting
A demand study is only as good as the discounting methodology applied at the end. Unfortunately, this is an often-overlooked aspect of many studies we’ve seen in the past. Demand+ is built to bring the discounting conversation to the forefront as a key component of study design.
We can all agree that study results tend to be more optimistic than what we see in the real-world. While one could take a static figure of 20-50% as the discount factor applied across the board, we feel that there are additional approaches to consider.
- Discounting based on analogs both in and out of category, and time to peak share
- Discounting based on price, knowledge, or barriers to access
- Discounting based on preference shares built for the products in the current market vs. actual market share from secondary research
With any of the approaches above, Demand+ is designed to deliver the most accurate, fine-tuned, and refined share estimations for your product and key competitors.
Interested in Learning More About Demand+?
We’d be happy to schedule a call with Lissa and our team to learn more about your challenges and needs, walkthrough Demand+ in more detail, and address any questions.
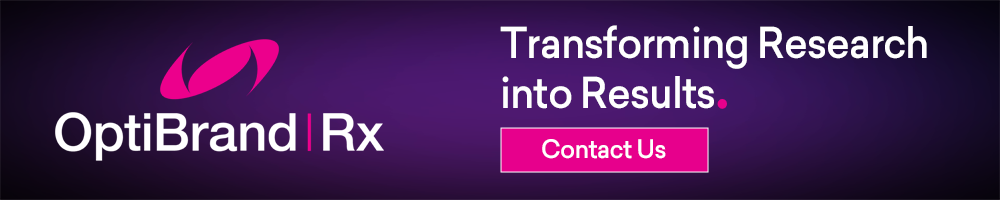